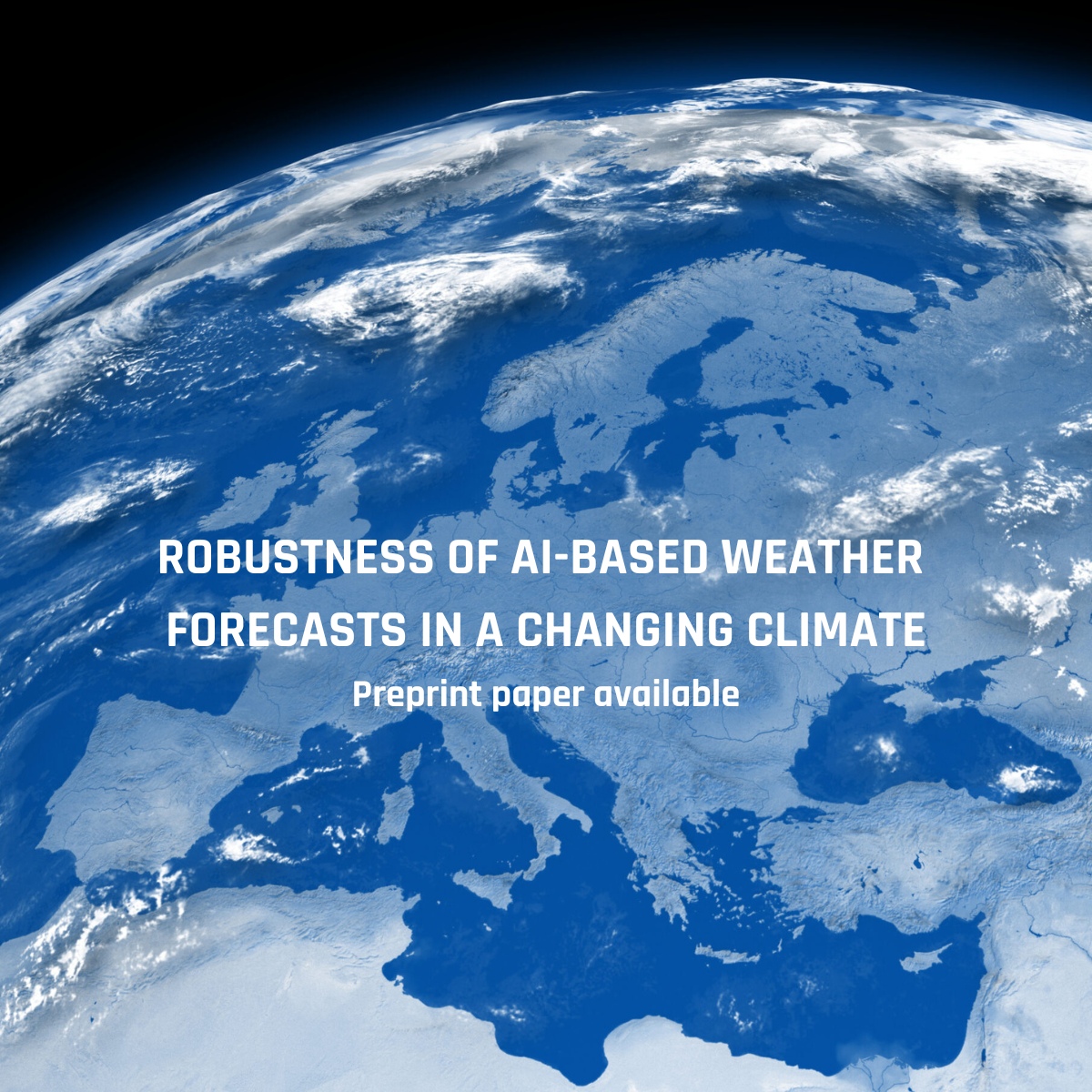
A preprint paper co-authored by scientists from ECMWF and the Alfred Wegener Institute, Helmholtz Centre for Polar and Marine Research (AWI) has explored the robustness of current Artificial-Intelligence-based weather models to run in possible future warmer worlds.
The authors, led by Thomas Rackow and Christian Lessig (both ECMWF) and Nikolay Koldunow (AWI), have put three state-of-the-art AI-based models, including ECMWF’s AIFS, to the test by performing 10-day forecasts in pre-industrial climate, in the present, and in a future climate 2.9ºC warmer than the pre-industrial average. The future climate was based on a scenario simulation with the IFS-FESOM model, performed in the H2020 project nextGEMS, by teams at ECMWF and AWI. The work was partly carried out in the framework of the Destination Earth initiative of the European Commission, in which ECMWF is responsible for implementing two Earth system Digital Twins and the underlying software infrastructure, the Digital Twin Engine, together with many partners throughout Europe.
The three AI-based models have shown critical out-of-distribution capabilities in terms of forecast skill when being applied in pre-industrial and hypothetical future conditions (see Gallery), opening the door to a wide range of applications in climate science.
Gallery: Skill of data-driven 10-day weather forecasts for 2m temperature in conditions representing preindustrial, present-day, and future +2.9K climate states, for AIFS, GraphCast, and Pangu-Weather. Root-mean square error (RMSE, in K) for all models, weighted by the cosine of latitude in a, 1955, a proxy-year for pre-industrial times, b, in 2023, representing present-day climate, and c, in 2049, the +2.9K warmer world. d, Mean bias [K] for all models in 1955, e, in 2023, and f, in 2049. In 1955, Pangu-Weather cools further while the other two models show a warming. In 2023, GraphCast and AIFS show very little bias, similar to ECMWF’s operational IFS forecast after 10 days, while Pangu-Weather consistently cools. In 2049, positive and negative biases for GraphCast balance while the other two models are characterised by a cold bias. All RMSE and bias evolutions in 1955, 2023, and 2049 are averaged over the daily forecasts.
At the same time, two of the models showed a cold bias for future warmer conditions, driven by the fact that the models are trained in the (colder) current climate on the ERA5 reanalysis produced in the framework of the Copernicus Climate Change Service at ECMWF. Similarly, two models show a warm bias for pre-industrial conditions, indicating that climate applications will still need to account for these current limitations (see Figure 1).
Due to the strong link between weather and climate modelling, physics-based weather forecast models have been used for several climate applications before. Despite the current limitations shown by the AI-based forecasts, which are partly due to missing ocean-sea ice and land surface information in the training data set, the new experiments have shown that AI-based models can recognise and handle new data which is different than the data used for their training, which is a critical prerequisite for applications planned in DestinE. With the demonstrated generalisation capabilities, the fast and inexpensive AI-based models now have the potential to be used for climate applications.
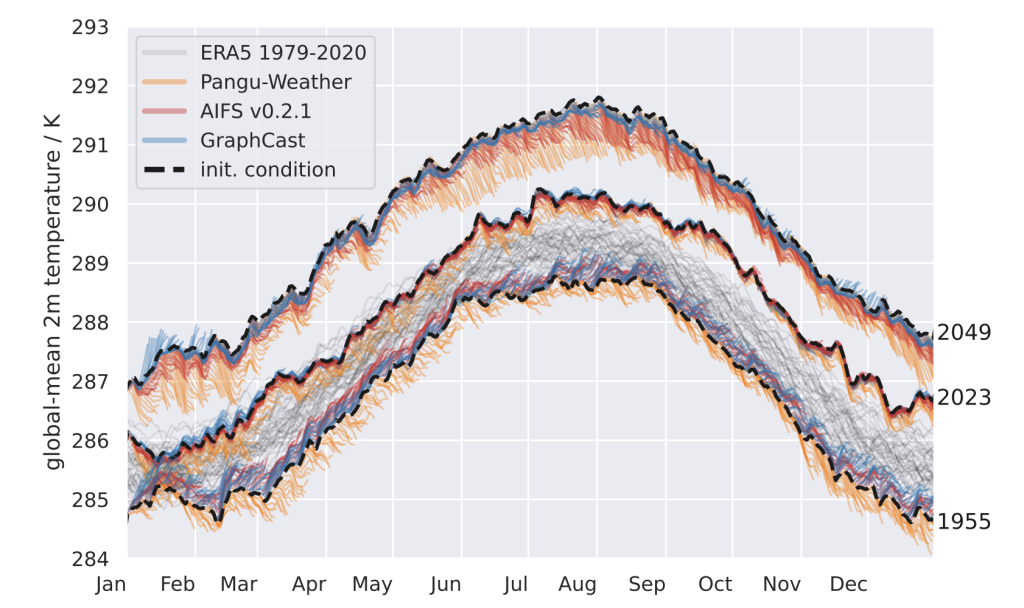
For example, they could be used to create an ensemble of future weather states and help to estimate uncertainty around km-scale climate projection runs, such as those performed by the DestinE Climate Change Adaptation Digital Twin. However, this approach needs some further work to retrain and recalibrate the models for this purpose, in order to reduce the observed drifts in future conditions.
Data-driven weather forecasting models could also be used as dynamic interpolation engines to reconstruct in-between states from a sparse set of output fields. This could help to reduce the storage requirements for climate simulation data, which become substantial in km-scale simulations such as those performed in DestinE.
The new findings highlight the potential of AI-based models to transform established approaches to climate projection and uncertainty quantification in the near future, providing a powerful complement to conventional physics-based models.
Read the abstract on Arxiv. We want to note that the study has not yet undergone peer-review, meaning that the findings are provisional. While the conclusions may undergo some adjustments, they are expected to remain largely consistent.
Destination Earth is a European Union-funded initiative launched in 2022, with the aim to build a digital replica of the Earth system by 2030. The initiative is being jointly implemented under the leadership of DG CNECT by three entrusted entities: the European Centre for Medium-Range Weather Forecasts (ECMWF), responsible for the creation of the first two ‘digital twins’ and the ‘Digital Twin Engine’, the European Space Agency (ESA) responsible for building the ‘Core Service Platform’, and the European Organisation for the Exploitation of Meteorological Satellites (EUMETSAT), responsible for the creation of the ‘Data Lake’.
We acknowledge the EuroHPC Joint Undertaking for awarding this project strategic access to the EuroHPC supercomputers LUMI, hosted by CSC (Finland), and the LUMI consortium, Marenostrum5, hosted by BSC (Spain) Leonardo, hosted by Cineca (Italy) and MeluXina, hosted by LuxProvide (Luxembourg) through a EuroHPC Special Access call.
More information about Destination Earth is on the Destination Earth website and the EU Commission website.
For more information about ECMWF’s role visit ecmwf.int/DestinE
For any questions related to the role of ECMWF in Destination Earth, please use the following email links: