Overview
DE_371
Overview
The objective of this contract is the application of deep learning methods, specifically generative machine learning techniques such as Variational AutoEncoders (VAEs), Generative Adversarial Networks (GANs), normalizing flows, and denoising diffusion models, to improve weather forecasting.
The contract involves the generation of large ensembles of physically realistic weather scenarios and temporal downscaling for existing weather forecasts using these machine learning techniques. This is executed through a collaborative effort involving researchers and engineers from MET Norway, Météo-France, and SMHI, with expertise in Numerical Weather Prediction (NWP) and GAN-based ensemble generation.
The project focuses on two main areas: further development and testing of the GAN-based ensemble approach and adapting the GAN approach for temporal downscaling. The methods will be applied to NWP data of various spatial resolutions and domains to assess their performance in terms of probabilistic forecasting, physical consistency, realism, and computational requirements. The project aims to deliver machine learning solutions that can be integrated into other projects and will provide code repositories, documentation, and containers for ease of use. The ultimate goal is to incorporate the GAN approach into the DestinE Digital Twin Engine (DTE) workflow for weather forecasting.
Provider
The provider for this contract is Norwegian Meteorological Institute
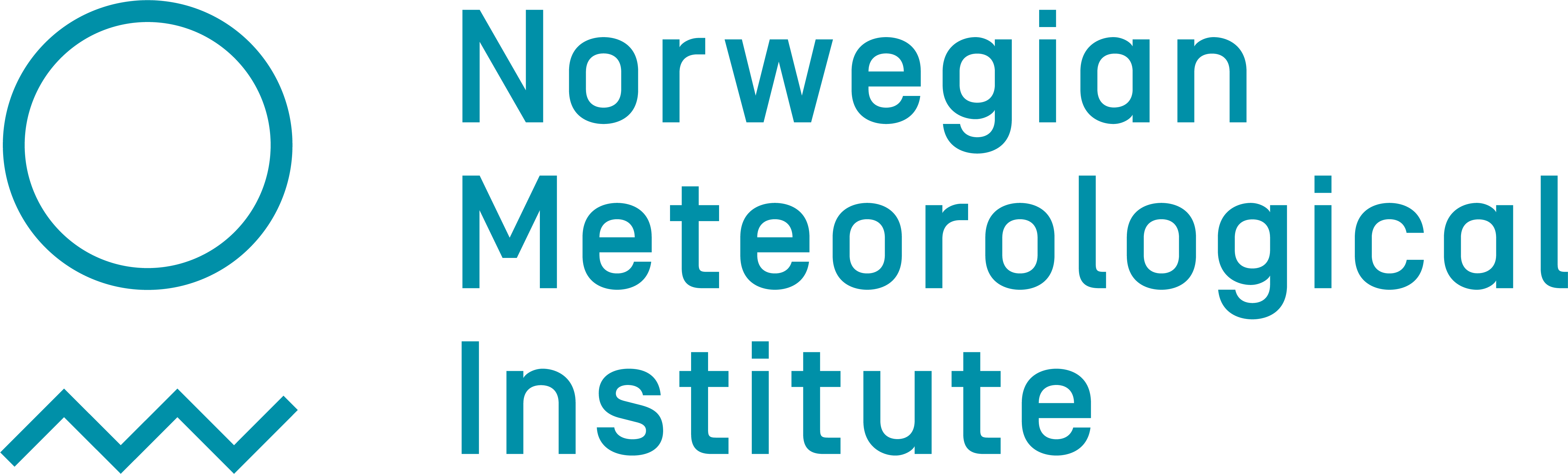
Subcontractors

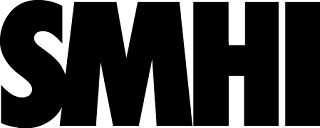