Facilitating their training is essential for advancing the next generation of data-driven models. Not only is this necessary for achieving the developments and integrations of a new generation of ML models within DestinE, but it supports the broader landscape of AI advancements in weather and climate applications across Europe. Although computationally demanding, these training activities are a key catalyst for progress.
EuroHPC in DestinE: a Premier Resource for AI Training
The EuroHPC supercomputers at the heart of DestinE are equipped with the latest accelerator technology, making them the ideal environment for AI training. These accelerators, such as GPUs, can perform massive numbers of calculations simultaneously, which is a strong asset for the demanding AI training tasks.
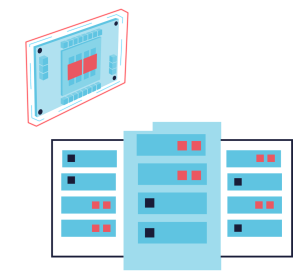
By providing cutting-edge computational performance, the EuroHPC supercomputers can help accelerate the training of ML models, enabling developers to iterate on models more quickly and unlock breakthroughs in AI capabilities. However, as the application of ML techniques for Earth-system modelling is still relatively new, their full exploitation requires the deployment of novel approaches for data management and of specific software.
Expanding software solutions for the new generation of AI models
Due to its complex decentralised environment and the unprecedented volume of data generated by the digital twins, DestinE has brought innovative data handling techniques into its architecture, for example through the Digital Twin Engine (DTE), one of DestinE’s main components. Among its various functions, the DTE ensures the seamless management of the vast data volumes produced by the digital twins. This advanced framework not only facilitates efficient data management but also provides a solid foundation for effectively leveraging both DestinE digital twin data and the EuroHPC supercomputers for training a new generation of ML models in DestinE.
The activities undertaken by ECMWF and its partners in DestinE’s second phase are further expanding the software solutions of the DTE, supporting the development of AI-driven weather and climate models, and their integration into supercomputing environments like those of EuroHPC.
For example, some of the main developments will enhance the Digital Twin Engine by embedding ML pipelines into its framework. The goal is to further develop its data handling and processing capabilities, ensuring they enable the development, training, and execution of ML/AI models on EuroHPC supercomputers by exploiting the DestinE digital twins data.
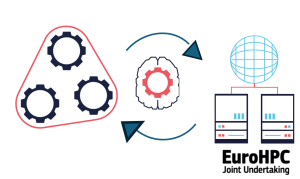
Key efforts include optimising GPU utilisation and parallelisation for more efficient data management, designing new pipelines and workflows, and integrating ML processes into the DestinE DTE production environment. This integration will streamline the configuration, execution, and monitoring of ML training and inference of the ML Earth-system components developed in DestinE.